*VDE 논문 리뷰를 위한 글 입니다! 궁금하신 점이 있다면 댓글로 달아주세요!
*이 논문에서는 Self-driving car에서 Distance estimation을 수행하는 딥러닝을 제시합니다.
VDE(ODD) paper: https://www.mdpi.com/2073-8994/14/12/2657
Vehicle Distance Estimation from a Monocular Camera for Advanced Driver Assistance Systems
The purpose of this study is to propose a framework for accurate and efficient vehicle distance estimation from a monocular camera. The proposed framework consists of a transformer-based object detector, a transformer-based depth estimator, and a distance
www.mdpi.com
VDE(ODD) our github: https://github.com/KyujinHan/Object-Depth-detection-based-hybrid-Distance-estimator
GitHub - KyujinHan/Object-Depth-detection-based-hybrid-Distance-estimator: We use our VDEmodel. Our purpose is that predict the
We use our VDEmodel. Our purpose is that predict the distance between car based on Deep-Learning. - GitHub - KyujinHan/Object-Depth-detection-based-hybrid-Distance-estimator: We use our VDEmodel. O...
github.com
Contents
2. Background Knowledge: DETR, GLPDepth, LSTM
Simple Introduction
기존의 자율 주행 자동차에서 사용되는 distance estimation은 ADAS(Advanced driver assistance systems)라는 기능을 이용하거나 LiDAR를 활용해서 같이 Deep learning과 적용하는 연구가 활발하였다.
그러나 우리는 오직 Deep learning framework만을 이용하여 Distance estimation을 진행하였고,
기존의 선행연구들보다 뛰어난 성능을 제시하였다.
어떻게 모델을 구성했는지 한번 알아보자!
Background Knowledge: DETR, GLPDepth, LSTM
DETR 논문 리뷰: https://kyujinpy.tistory.com/4
[DETR 논문 리뷰] - End-to-End Object Detection with Transformers
*DETR 논문 리뷰를 위한 글입니다! 궁금하신 점이 있다면 댓글로 남겨주세요. DETR paper: [2005.12872] End-to-End Object Detection with Transformers (arxiv.org) End-to-End Object Detection with Transformers We present a new method th
kyujinpy.tistory.com
GLPDepth 논문 리뷰: https://kyujinpy.tistory.com/5
[GLPDepth 논문 리뷰] - Global-Local Path Networks for Monocular Depth Estimation with Vertical CutDepth
*GLPDepth 논문 리뷰를 위한 글입니다! 궁금한 점이 있다면 댓글로 질문주세요! GLPDepth paper: [2201.07436] Global-Local Path Networks for Monocular Depth Estimation with Vertical CutDepth (arxiv.org) Global-Local Path Networks for M
kyujinpy.tistory.com
DETR과 GLPDepth, LSTM에 대한 구조 이해가 필요합니다!
DETR과 GLPDepth는 구조이해까지는 아니더라도, 어떠한 역할을 하는지 이해하고 있어야 하고,
LSTM에 대한 구조 이해는 있어야 논문을 쉽게 이해할 수 있다는 것을 말씀드립니다..!
+) (저의 블로그에서는 CNN, RNN와 같은 기본적인 모델(?)에 대한 논문 리뷰는 없습니다..ㅠㅠ)
Method
Model의 전체적인 구조는 이렇다.
1. Image를 input받으면 DETR과 GLPDepth에 넣어준다.
- DETR에서는 class, object의 bounding box 좌표를 출력한다.
- GLPDepth에서는 image의 depth을 만든다.
2. Depth map 위에 bounding box를 overlapping한다.
3. Overlappning 된 bounding box를 기준으로 각 object의 depth 통계지표를 계산한다.
4. Bounding box, class information, depth의 통계 values를 LSTM에 넣어서 각 객체의 real distance를 계산한다.
+) DETR과 GLPDepth의 structure이다.
+) 자세한 구조와 설명은 위의 논문 리뷰글에서 볼 수 있다.
+) 우리가 이용한 distance estimator(LSTM)의 구조는 이러하다.
+) LSTM과 뒤에 FFN을 붙여서 Real distance을 예측했다.
+) 우리는 딥러닝과 머신러닝을 비교하기 위해서, Distance estimator의 구조를 LSTM뿐만 아니라 RandomForest, XGBoost를 이용하였다.
Data Preprocessing
우리가 데이터셋의 bounding box coordinates를 이용할 때, IOU를 계산해서 일정 부분의 object 정보는 제거했다.
그 이유는 위의 사진 처럼, 우리는 bounding box를 이용해서 depth의 평균 값을 object에 mapping 시키는데,
bounding box가 앞에 있는 객체와 뒤에 있는 객체가 서로 겹치면 bounding box안의 depth의 평균값이 유사해지는 현상이 일어났다.
따라서 이 현상을 방지하기 위해서, 70% 이상 IOU가 나오면, 뒤에 있는 객체를 제거하였다.
Depth estimator을 학습할 때 이용했던 데이터셋의 Bounding box coordinates을 만들 때,
실제 GroundTruth의 bounding box의 라벨과 DETR이 예측한 bounding box의 label을 euclidean distance를 사용하여서 기존의 데이터를 DETR의 값으로 replace 하였다.
이 과정에서 잘못 matching된 label를 제거하였다.
Result
- 전체적인 성능은 LSTM이 뛰어났고, Car의 distance estimation 성능은 XGBoost가 우월했다.
- 우리가 만든 bounding box의 정보는 GroundTruth처럼 100% 정확한 답은 아니다. 그러나 우리 모델의 구조가 testing을 할 때도, DETR에서 나오는 bounding box를 이용하기 때문에, DETR의 bounding box를 이용했을 때 성능이 더 좋다.
- 기존의 ADAS와 LiDAR을 이용해서 Distance estimation을 진행한 모델보다 우리의 모델이 성능이 우월하다는 것을 알 수 있다.
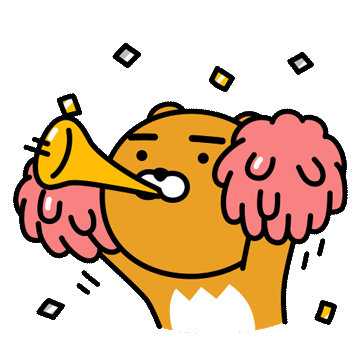
- My first SCI 논문! (당시 학부 3학년)
- 2023.01.09 Kyujinpy 작성.
'AI > CV project' 카테고리의 다른 글
[KO-stable-diffusion-anything] - 한국어 기반의 stable-diffusion-disney와 KO-anything-v4-5 (0) | 2023.08.16 |
---|---|
[OpenFlaminKO] - Polyglot-KO를 활용한 한국어 기반 MultiModal 도전기! (0) | 2023.08.16 |
D-NeRF를 이용한 Real dataset(real scene video) 학습하기 (5) | 2022.12.27 |
'Colmap' implementation method in only python scripts (0) | 2022.12.23 |
[D-NeRF 코드 분석] - D-NeRF: Neural Radiance Fields for Dynamic Scenes (0) | 2022.12.23 |